AI models have become increasingly powerful with time, but it’s still not completely understood how exactly they work. A startup is looking to change that.
San Francisco based AI startup Goodfire has raised $50 million in its Series A funding round. The round was led by Menlo Ventures with participation from Lightspeed Venture Partners, Anthropic, B Capital, Work-Bench, Wing, South Park Commons, and other investors. The company also announced an interpretability product named Ember, which would help researchers understand what makes AI models tick.
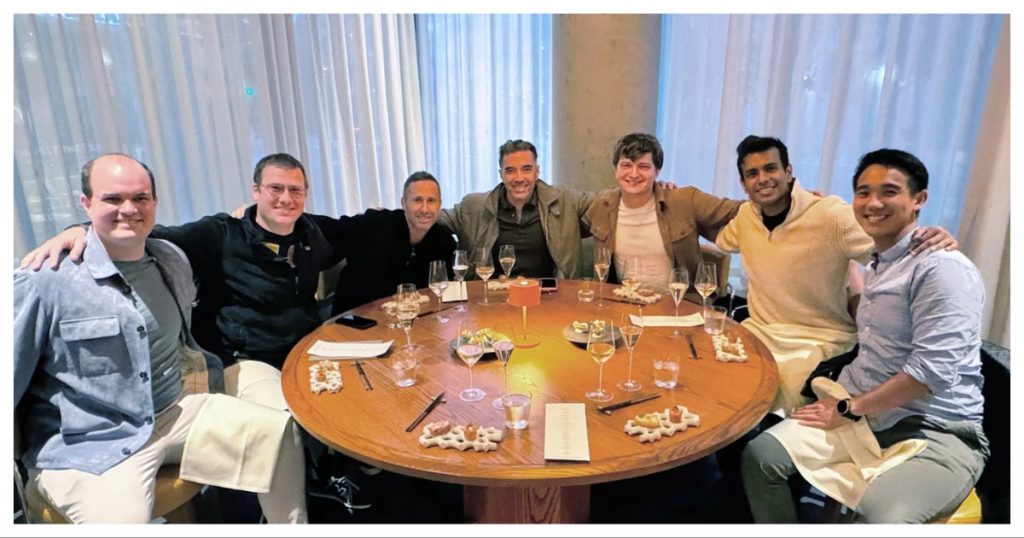
“Nobody understands the mechanisms by which AI models fail, so no one knows how to fix them,” said Eric Ho, co-founder and CEO of Goodfire. “Our vision is to build tools to make neural networks easy to understand, design, and fix from the inside out. This technology is critical for building the next frontier of safe and powerful foundation models.”
“AI models are notoriously nondeterministic black boxes,” said Deedy Das, investor at Menlo Ventures. “Goodfire’s world-class team—drawn from OpenAI and Google DeepMind—is cracking open that box to help enterprises truly understand, guide, and control their AI systems.”
Goodfire has launched a new product named Ember, which uses the latest in mechanistic interpretability research to decode a model’s thoughts and gives direct programmable access into the model’s internal representations. In a demo, the product was able to understand the underlying representations of an AI-generated image of lions wearing Santa hats to show which parts corresponded to lions and which parts to the hats. The product then lets users to “draw” these representations, and can change the picture to add more lions and more Santa hats.
The company’s work on language models sounds even more interesting. “We’ve also interpreted language models to enable neural programming. Language models will deny that they’re conscious, but if you do brain surgeries on these models and turn up their consciousness neurons, they’ll then change their tune,” Goodfire says.
These approaches could be useful for researchers who’re trying to figure out how exactly AI models work. Unlike regular computers, which are deterministic, AI models are composed of billions of neurons, and their unique activations to human inputs lead to their outputs. These outputs aren’t always the same, or entirely predictable. But if companies like Goodfire can peek under the hood and figure out what exactly is going on inside AI models, it can not only help understand and fine-tune current models, but also pave the path for new approaches and techniques in building even stronger AI.