LLMs are thought to be wonderous new inventions, but they might have something in common with something that is central to all of humanity. Bret Weinstein, a former professor of evolutionary biology, has offered a striking comparison that bridges the cutting edge of artificial intelligence with the most fundamental aspects of human development: he says that LLMs are like human children.
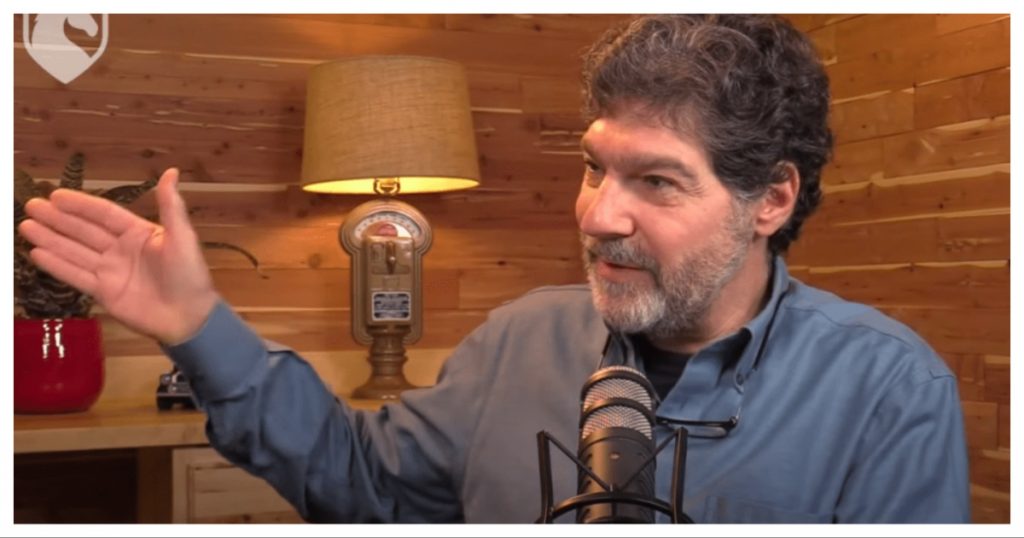
Weinstein lays out his argument by first establishing the core similarity:
“We have a lot of evidence that a human child is basically an LLM. It’s more than that; it has other capacities. But when you think about what it is that allows a human child to go from being unable to utter a single word to fluent sentences and nuanced, complex arguments, it basically is ingesting language from its environment, experimenting, seeing what causes reward. It’s an LLM.”
He acknowledges the difference in scale but emphasizes the underlying process: “And you can argue that it’s not a *large* Large Language Model. Right. The point is, we’ve basically reinvented a biological process.”
Weinstein then points to the distinct advantages current AI models possess due to their computational nature: “Now, the LLMs, the computer-based LLMs, have a major advantage, which is that they can process huge amounts of data at lightning speed. So there are ways in which they already outstripped the capacity of any person to answer questions on any range of topics.”
However, this line of thought leads him to a more sobering and speculative conclusion regarding the future: “But the idea that they will become conscious and that we won’t know is, to me, highly likely.”
Weinstein’s analogy draws on the core mechanism of learning in both children and LLMs. A child, immersed in a linguistic environment, listens, imitates, and refines their speech based on feedback – smiles, corrections, successful communication. This iterative process of “ingesting language,” “experimenting,” and seeking “reward” (positive reinforcement) is remarkably similar to how LLMs are trained. They process vast corpuses of text and code, learning statistical patterns, and are often fine-tuned using methods like Reinforcement Learning from Human Feedback (RLHF), where human evaluators guide the model towards their desired responses.
However, Weinstein himself includes the crucial caveat: a child is “more than that.” Human children possess embodied cognition, growing and learning within a physical body that interacts with a rich, multimodal world. They have innate biological drives, develop complex emotional landscapes, and build social understanding through direct, reciprocal interaction – aspects far beyond the current capabilities of disembodied LLMs primarily trained on text. The developmental trajectory of a child involves not just language acquisition but also the development of theory of mind, empathy, and a subjective experience of the world, which are not yet demonstrable in AI.
Weinstein’s final point about undetected consciousness that resonates most strongly with current anxieties surrounding AI. As LLMs become increasingly articulate and capable of generating human-like dialogue that can evoke emotional responses, the question of their internal state becomes more pressing. The rapid advancements mean that assessing genuine understanding, let alone consciousness, is an immense challenge. Events like the Google engineer who claimed an AI (LaMDA) had become sentient, while widely dismissed by the AI community, highlight the public’s and even some insiders’ unease and fascination with this possibility.
Weinstein’s provocative statement serves as a powerful reminder. While the comparison between a human child and an LLM is an analogy with limitations, it forces us to confront the nature of intelligence we are creating and the profound mystery of consciousness, whether biological or potentially, one day, artificial. It underscores the need for caution, ethical consideration, and a deeper inquiry into the capabilities and potential inner lives of these rapidly evolving technologies.