Researchers have come up with clever ways to train LLMs over the last few years, and they’re making similar breakthroughs in robotics.
NVIDIA’s AI Director, Jim Fan, recently shared some interesting progress in robot training. He details how advancements in simulation have dramatically accelerated the learning process for complex robotic tasks, hinting at a future where robots can be rapidly trained for a variety of real-world applications. The implications of his words are profound, suggesting a new era of adaptable and skilled robots.
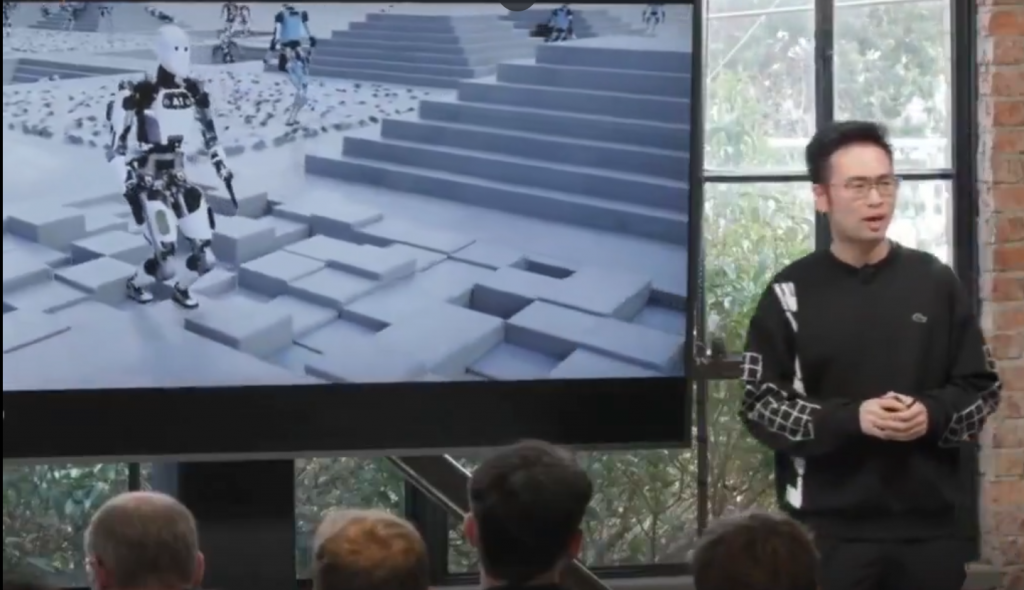
Fan described a technique through which robots don’t need to be trained in the physical world, but are instead trained digitally through simulations. While a physical robot can only be trained for 24 hours in a day, thousands of such simulations can be run in parallel on computers. Once the robot is trained digitally, its learned are passed on to the physical robots. These robots can then immediately replicate thousands of hours worth of learning in the real world. “These humanoid robots went through 10 years worth of training in only two hours of simulation time to learn walking,” Fan said.
“And then you can transfer that, and it doesn’t matter what the embodiment is. As long as you have the robot model, you simulate it, and you can do the walking,” he added.
“And can we do more than walking? So as we are controlling our body, you can try any pose that you want, track any key point, follow any velocity vector that you want. And this is called the whole body control problem of humanoid, and it’s really difficult, but we can train that right on 10,000 simulations running parallel,” he said. NVIDIA showed off robots doing Cristiano Ronaldo’s signature celebration after having been trained only digitally through simulations.
Fan said that this required a fraction of the size of the neural networks employed by modern LLMs. “And guess how big of the neural network it is required to do this? It is 1.5 million parameters, not billion. 1.5 million parameters is enough to capture the subconscious processing of the human body, the system one reasoning,” he said.
This ability to compress a decade of learning into a mere two hours of simulation time could mark a significant leap forward. Traditionally, training robots involved painstaking real-world trials, often limited by safety concerns, equipment fragility, and the sheer time required for a robot to accumulate sufficient experience. NVIDIA’s approach, leveraging high-fidelity simulation and parallel processing, circumvents these limitations, allowing robots to rapidly iterate and learn complex behaviors.
The “zero-shot” transfer to real-world robots is particularly impressive. It indicates that the simulated environment is accurately capturing the physics and dynamics of the real world, allowing robots to generalize their learned skills without needing further fine-tuning. This could help robots scale extremely quickly once their training is deemed sophisticated enough to be used in the real world. The relatively small size of the neural network – just 1.5 million parameters – is also noteworthy. This suggests that efficient algorithms and optimized architectures are playing a crucial role in enabling complex behaviors with limited computational resources. This has major implications for deploying robots in resource-constrained environments and potentially reducing the energy footprint of robotic systems. It’s an exciting time for AI and robotics, and NVIDIA seems to be one of the companies leading the charge.