There have been several accidental discoveries in science, including penicillin and the microwave oven, and modern LLMs might fall somewhat in the same ballpark.
OpenAI CEO Sam Altman has said that the employee who built GPT-1, the first version of ChatGPT, didn’t quite understand how or why it worked, but was impressed with the results. As the teams worked further on GPT-1, they realized that making the models bigger improved their performance, and finally released GPT-3 as ChatGPT in November 2022. This was the event that’s set of the AI revolution we’re in today.
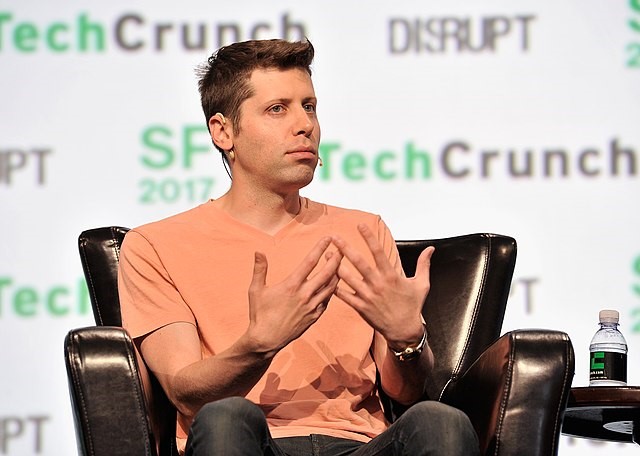
“You see this in a lot of the history of technology,” Altman said in a conversation with Bill Gates, “where someone makes an empirical discovery, they have no idea what’s going on, but it clearly works. And then, as the scientific understanding deepens, they can make it so much better,” he added.
“In our case, we had, you know, someone that was… the guy that built GPT-1 sort of did it off by himself and saw this. And it was impressive.”
However, he admitted a crucial gap in their initial understanding: “But, you know, (he had) no deeper understanding of how it worked or why it worked.” But this initial lack of complete understanding didn’t deter the team. “And then we got these scaling laws, we could predict how much better it was going to be. That was why when we told you we could do that demo, we were pretty confident it was gonna work. We hadn’t trained the model, but we were pretty confident.”
He concludes by noting this empirical approach ultimately paved the way for more rigorous scientific inquiry: “And that has led us to a bunch of attempts and better and better scientific understanding of what’s going on. But it really came from a place of empirical result first.”
Altman’s remarks provide a compelling glimpse into the sometimes haphazard nature of technological progress. The fact that such a transformative technology emerged from a place of “messing around” underscores the importance of fostering an environment of experimentation and embracing unexpected results. While the initial success of GPT-1 might have been based on observation rather than deep theoretical understanding, the subsequent focus on scaling laws and scientific investigation allowed OpenAI to refine and significantly improve the models, leading to the release of ChatGPT and the current surge in AI capabilities. This iterative process, moving from empirical observation to scientific understanding, is a crucial lesson for future development in the field of AI and beyond — and highlights the importance of both intuitive experimentation and rigorous scientific analysis in driving technological breakthroughs.