There has been much talk about how AI could recursively self-improve in the coming years, but it appears that Google researchers have already seen evidence of this in their own labs.
David Silver, a prominent researcher at Google DeepMind and a key figure behind AlphaGo, the AI that famously defeated a world champion Go player, recently shared some interesting insights on the development of Reinforcement Learning. He revealed that DeepMind has created an AI system capable of designing its own reinforcement learning algorithms. What’s more, these AI-generated algorithms have surpassed the performance of algorithms meticulously crafted by human experts over many years. This development could represent a significant leap towards self-improving AI and carries profound implications for the future of the field.
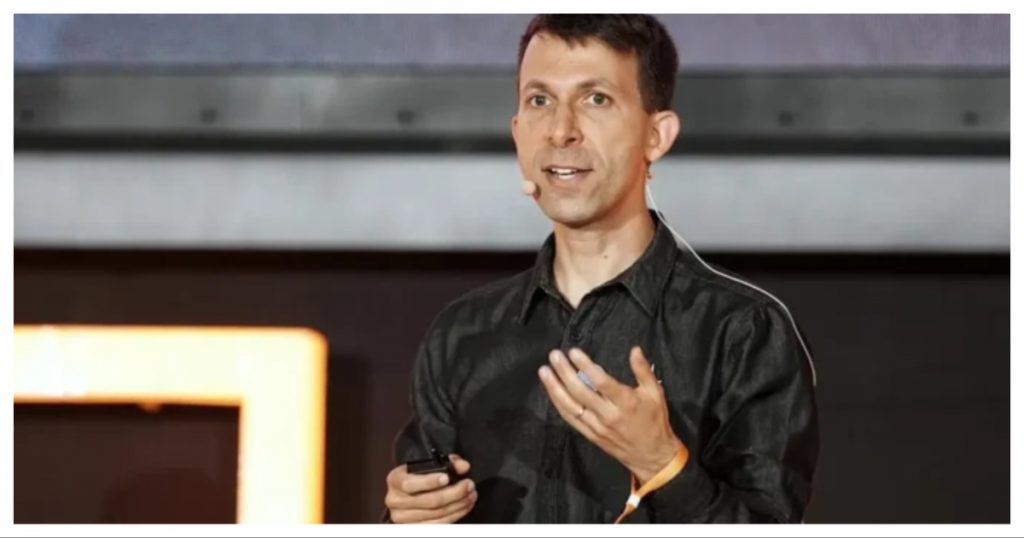
“Can AI design its own reinforcement learning algorithms?” the interviewer asked him. “Well, funnily enough, we have actually done some work in this area, which we actually did a few years ago, but is coming out now,” he replied.
“What we did was actually to build a system that, through trial and error, through reinforcement learning itself, figured out what algorithm was best at reinforcement learning.”
This is where the concept becomes truly mind-bending: “It literally went one level meta,” Silver emphasized, “and it learned how to build its own reinforcement learning system.”
The results were even more striking: “Incredibly, it actually outperformed all of the human reinforcement learning algorithms that we’d come up with ourselves over many, many years in the past.”
Reinforcement learning (RL) is a type of machine learning where an agent learns to make decisions by interacting with an environment, aiming to maximize a cumulative reward. Unlike supervised learning, which relies on labeled data, RL operates through trial and error: the agent takes actions, observes the outcomes, and adjusts its strategy based on feedback in the form of rewards or penalties. This approach mirrors how humans learn from experience, making RL a close proxy to human intelligence. Silver seemed to be suggesting that their AI agent, through trial and error, was able to create its own algorithms for rewards and penalties to improve its performance, and outperformed the algorithms that the human researchers had created.
The implications of this breakthrough are immense. If AI can design algorithms superior to those created by humans, it opens the door to accelerated progress in countless fields. Imagine AI optimizing algorithms for drug discovery, materials science, climate modeling, or even designing more efficient AI systems themselves. This recursive self-improvement loop, where AI bootstraps its own development, could lead to an exponential growth in AI capabilities.
However, this development also raises important questions about control and oversight. As AI systems become more sophisticated and autonomous in their development, it becomes crucial to ensure they remain aligned with human values and goals. These are exciting times in AI, but researchers and companies would do well to keep a close eye on some emergent properties that now seem to be developing.