There have been concerns around how AI labs were running out of data to train AI models, but it appears that AI models themselves could first generate fresh training data — which could be used to train more sophisticated AI systems.
Aidan Gomez, CEO of Cohere and a co-author of the seminal “Attention is All You Need” paper that introduced the transformer model architecture, recently offered compelling insights into the future of AI training data. In a discussion, Gomez highlighted the growing reliance on synthetic data, particularly in specialized fields where obtaining real-world data is prohibitively expensive or logistically challenging. His comments shed light on a critical shift in the AI landscape, where synthetic data is no longer a mere supplement but is rapidly becoming the primary fuel for increasingly sophisticated models. He argues that while human data remains essential, its cost and scarcity necessitate innovative solutions.
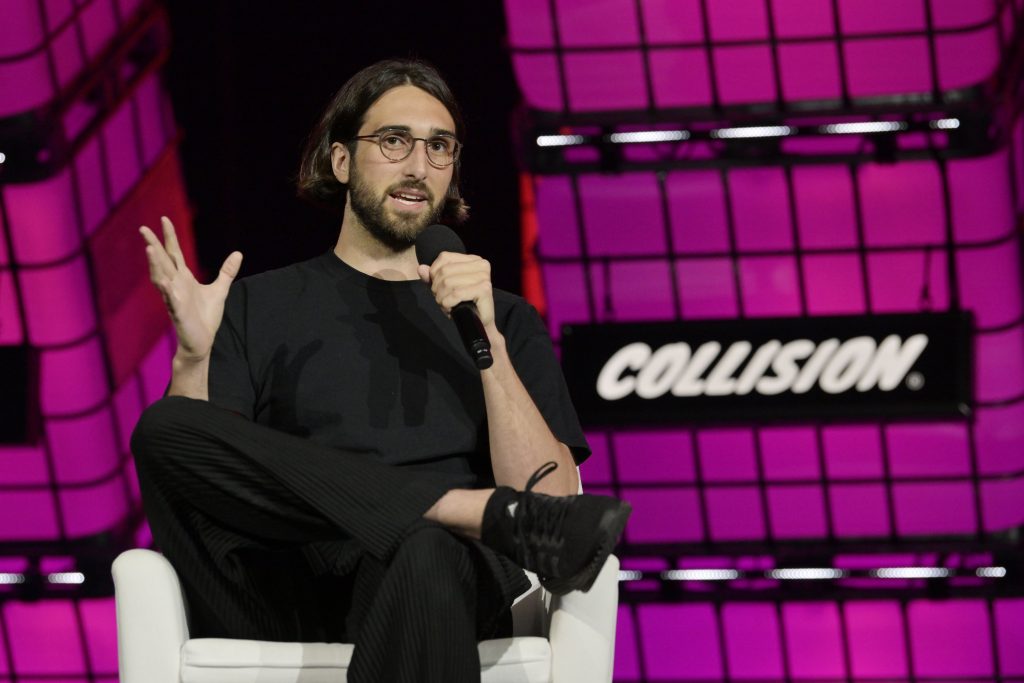
“On the data generation side,” Gomez explains, “it’s too expensive (to find specialized data). Yes, we definitely still need human data. But it’s too expensive to go find 100,000 doctors and have them teach the model to do medicine. That is not a viable strategy.” He contrasts this with the earlier phase of model development, which relied on average people and not specialists like doctors. “It was a viable strategy to teach the model general things, about how to converse, chit-chat, that type of thing to find 100,000 average people, and they can teach the model to do that stuff.”
But finding 100,000 doctors is a lot harder than finding 100,000 regular people. “So we’ve had to become much more creative. But teaching the model to chit-chat and do all that stuff unlocked a certain degree of freedom in terms of synthetic data generation, which we can then apply to specific domains like medicine.” This allows for a more targeted use of valuable human expertise: “We can use a much smaller pool of human data. You know, maybe I go to 100 doctors and get them to provide me some lessons and teach my model. And then I use that pool of, like, known good, very trustworthy data to generate a 1,000-fold synthetic lookalike.”
Gomez also addresses the challenges of verifying synthetic data quality: “In verifiable domains like code and math, it’s way easier because you can check the results and use that to filter the synthetic data and pull out the garbage and, you know, find the gold. In other domains, it becomes much more complex, but it’s still viable.” He concludes with a revealing statistic about Cohere’s current data usage: “So at this stage, I would say in terms of, like, the overall data that Cohere is generating for a new model, an overwhelming majority is synthetic.”
Many top AI experts have voiced concerns about the limited availability of data to train models on. Elon Musk has said that models have already used up all available data. SSI’s Ilya Sutskever has said that data is the fossil fuel of AI, and it will one day get exhausted. But as Cohere’s Aidan Gomez says, there could be creative ways to come up with synthetic data that can continually be used to train new models. And it appears that existing AI models could help generate this synthetic data to train bigger and more capable systems.