The basis of much of Artificial Intelligence was the human brain — neural networks, in particular, were modeled on how the human brain worked — but the field is now helping understand the human brain better.
Dario Amodei, CEO of Anthropic, the AI research company behind the Claude series of language models, recently highlighted a fascinating shift in the relationship between neuroscience and artificial intelligence. In a discussion after the release of Claude’s latest series of models, Amodei pointed out that while the initial impetus for AI development came from trying to emulate the human brain, the flow of inspiration is now reversing. This is largely due to advancements in AI interpretability, which allows researchers to “see inside” complex AI models and then use the results to understand the human brain.
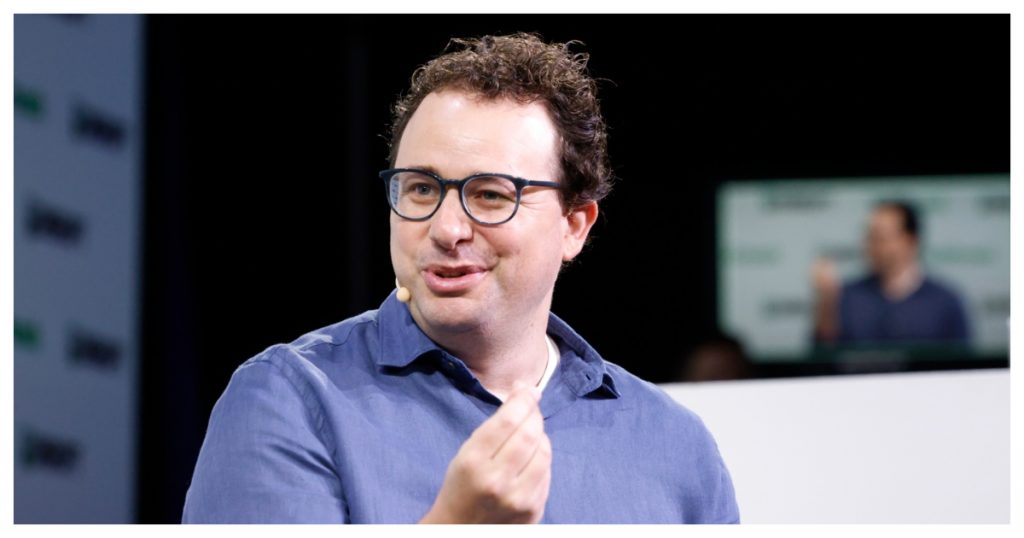
“I think 10 years ago many people thought that neuroscience would tell us about how to do AI, and indeed there are a number of former neuroscientists in the field. I’m not the only one,” Amodei said. He acknowledged the early influence of neuroscience, but also admitted the limitations of directly translating neuroscientific findings into AI architectures. “At a high level, there’s some inspiration, but I wouldn’t say I’ve said, ‘Oh, this is how the thing we know from the hypothalamus we can use for making these models.’ It’s all been pretty much from scratch.”
The real turning point, according to Amodei, is the ability to understand the inner workings of AI models themselves. “Interestingly, things have gone the other way more, which is that using interpretability, we’re able to see inside models, and although, of course, they’re not made in exactly the same way the human brain is, at the superficial level there are a lot of differences, a lot of the conceptual patterns we have found inside models sometimes they then get replicated in neuroscience research.”
He even provided a specific example of this reverse inspiration. “There was something about like high/low frequency detectors in vision that was found by interpretability via one of the people on Chris Olah’s team, and then a couple years later, a neuroscientist actually replicated it in animal brains.” This anecdote powerfully illustrates how AI, originally conceived as a mimicry of the brain, is now offering valuable insights back into it.
Amodei’s comments echo a growing trend within the AI research community. As AI models become increasingly complex, the need to understand how they arrive at decisions has become paramount. This has spurred the development of “interpretability” tools and techniques, which allow researchers to probe the internal representations and processes within these models. These insights, it turns out, can also provide valuable hypotheses for neuroscientists studying the brain. Ultimately, the bidirectional relationship between AI and neuroscience promises to accelerate progress in both fields, leading to more powerful and safer AI systems, as well as a more profound understanding of the human brain.