The rapid growth of research publications and data in recent years has created a paradox for researchers: while access to information is unprecedented, managing and analyzing this vast amount of material has become increasingly challenging. Artificial Intelligence (AI) has emerged as a transformative tool to address this issue, helping researchers efficiently analyze and interpret complex data while reducing the time spent on labor-intensive tasks. This article explores the many ways AI can assist researchers in streamlining their workflows, enhancing productivity, and generating meaningful insights.
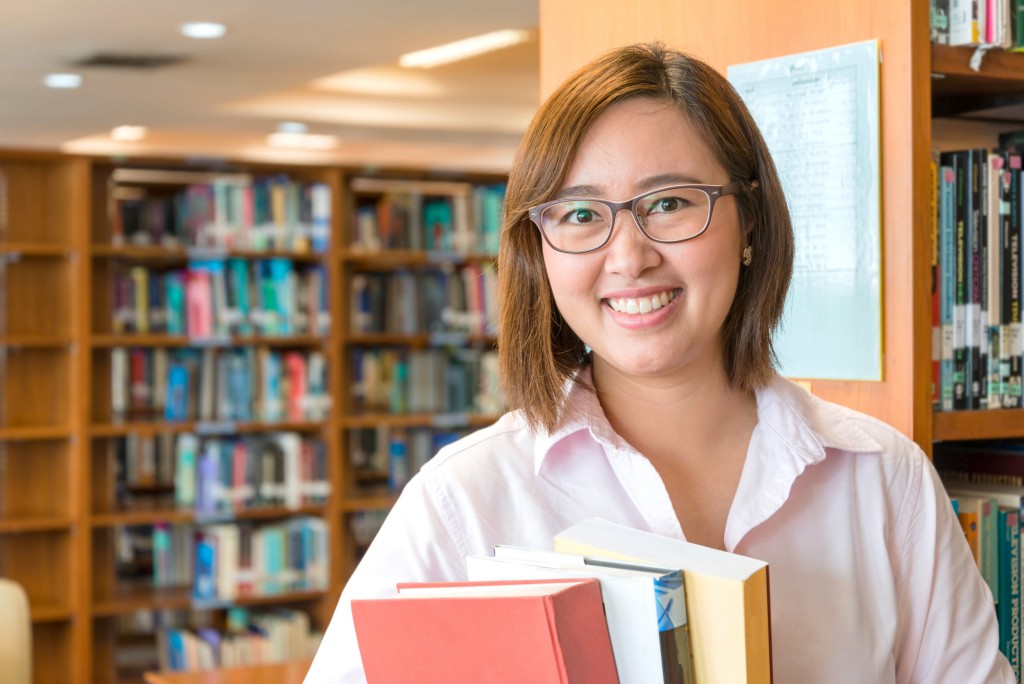
The Research Challenge: Information Overload
The exponential growth of scientific literature and data poses a significant challenge to researchers. A single field can produce thousands of articles and datasets annually, making it difficult to stay current or pinpoint relevant findings. This information overload often results in researchers spending an inordinate amount of time sorting through documents, extracting key insights, and synthesizing data into actionable conclusions.
AI offers a powerful solution to this bottleneck by automating repetitive tasks, enhancing data interpretation, and providing intuitive tools to navigate the ever-expanding research landscape.
How AI Can Help Researchers
1. Automated Literature Review
Conducting a comprehensive literature review is one of the most time-consuming stages of research. AI tools such as natural language processing (NLP) models can:
- Search and Summarize: AI-powered platforms like Semantic Scholar or tools integrated into academic search engines help researchers quickly identify relevant papers, summarize key findings, and highlight critical methodologies or conclusions.
- Topic Clustering: AI can group papers into themes or topics, enabling researchers to explore trends and gaps in the literature with minimal effort.
By reducing the hours spent manually searching and annotating, researchers can allocate more time to analysis and experimentation.
2. Data Analysis and Pattern Recognition
Large datasets are increasingly common in disciplines such as genomics, climate science, and social sciences. Traditional methods of analyzing such data are often time-intensive and prone to human error. AI steps in by:
- Processing Large Data Volumes: Machine learning algorithms excel at identifying patterns, trends, and outliers in complex datasets.
- Real-Time Insights: AI can deliver real-time analytics, making it easier to adapt hypotheses and strategies during ongoing research.
- Predictive Modeling: By training on historical data, AI can predict future trends or outcomes, allowing researchers to explore “what-if” scenarios efficiently.
3. Enhancing Research Writing
Once the data has been analyzed, researchers face the challenge of communicating their findings effectively. AI tools designed for academic writing, such as Grammarly and ChatGPT, provide:
- Language Enhancement: Refining grammar, sentence structure, and vocabulary to produce polished manuscripts.
- Content Organization: Suggestions for logical flow and coherence in research papers.
- Citation Management: AI can automate the tedious process of formatting citations and references.
4. Extracting Information from PDFs and Research Documents
Manually sifting through lengthy PDFs or unstructured documents can be frustrating. AI-driven tools, allow researchers to interact with documents by asking direct questions and receiving instant answers. Features like keyword extraction, entity recognition, and contextual understanding make it easier to find specific information without reading every page.
5. Collaboration and Knowledge Sharing
AI tools facilitate collaboration by creating knowledge-sharing platforms that integrate with researchers’ workflows. For example:
- Automated Meeting Summaries: AI tools can summarize collaborative discussions and extract action items, saving time during team meetings.
- Translation Services: Language barriers are minimized with AI-powered translators, enabling researchers to access studies in multiple languages.
6. Hypothesis Generation
AI can assist in generating novel hypotheses by analyzing vast amounts of data and identifying correlations or relationships that may not be immediately apparent to humans. Tools like IBM Watson have demonstrated the ability to synthesize information from diverse sources, providing fresh perspectives on research problems.
Advantages of AI in Research
- Speed and Efficiency: Tasks that once took weeks can now be completed in hours.
- Accuracy: AI minimizes human error in data analysis and interpretation.
- Scalability: AI systems can handle increasing amounts of data with ease.
- Accessibility: Democratization of tools ensures that even smaller institutions or independent researchers can benefit.
The Future of AI in Research
The integration of AI into research is still in its early stages. Emerging technologies, such as quantum computing and advanced machine learning models, promise to further enhance AI’s capabilities. Future developments may include:
- Personalized AI Assistants: Tailored AI models for individual researchers or labs, offering bespoke insights and recommendations.
- Universal Knowledge Networks: Seamlessly integrating data from diverse disciplines to enable interdisciplinary research.
Conclusion
AI is fundamentally transforming how researchers approach their work, offering tools to manage information overload, analyze complex data, and communicate findings more effectively. By reducing the time required for repetitive tasks, AI allows researchers to focus on creativity, critical thinking, and innovation. As AI technologies continue to evolve, their potential to accelerate scientific discovery and solve complex global challenges will only grow, marking a new era in research efficiency and productivity.